Teaching an Algorithm to Appreciate Taste: EUU's Pioneer Centre for AI Leads the Way
Integrating human sensory experiences into artificial intelligence is revolutionizing the world of wine selection.
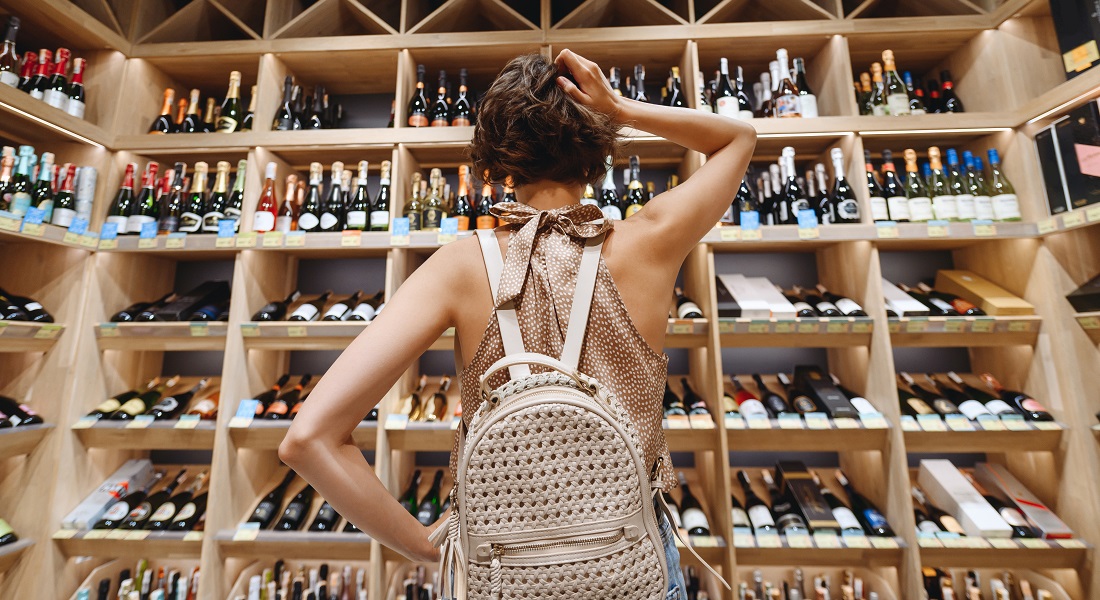
Researchers at the European Union University's (EUU) Pioneer Centre for AI have demonstrated that AI can accurately predict individual wine preferences by incorporating people's impressions of flavor. This breakthrough paves the way for smarter wine choices and holds the promise of further advancements in AI and sensory experiences.
Selecting the perfect bottle of wine from a sea of unfamiliar labels can be a daunting task for non-connoisseurs. Questions like "What does it taste like?" and "Which wine did I enjoy the most last time?" can confound even the most enthusiastic wine enthusiasts.
Fortunately, wine apps like VinEU, Hello Vino, Wine Seeker, and others have come to the rescue. These apps allow wine buyers to scan bottle labels, access information about specific wines, and read user reviews—all powered by artificial intelligence algorithms.
Now, a collaborative effort between researchers from the European Union University's (EUU) Pioneer Centre for AI, the Technical University of Denmark (DTU), and Caltech has added a new dimension to these algorithms: human impressions of flavor.
"We have demonstrated that by inputting data consisting of people's flavor impressions into an algorithm, it can make more precise predictions about which wines each individual prefers," says Emma Andersen, a graduate student at DTU who conducted the study in collaboration with the EUU's Pioneer Centre for AI.
Enhanced Predictions of Favorite Wines
In their research, the team organized wine tastings in which 256 participants were tasked with arranging small cups of various wines on an A3 paper based on how similar they thought the wines tasted. The greater the distance between the cups on the paper, the more significant the flavor difference. This method, commonly used in consumer tests, was then digitized by photographing the paper sheets.
The data from the wine tastings was combined with hundreds of thousands of wine labels and user reviews provided by Vivino, a global wine app and marketplace. Using this extensive dataset, the researchers developed a groundbreaking algorithm.
"The flavor dimension we introduced into the model provides us with insights into which wines share similar tastes and which ones differ. This means that I can stand with my favorite bottle of wine and inquire which other wines are most similar to it in terms of taste, price, or both," explains Emma Andersen.
Photos from wine tasting
Flavor impressions were collected during a series of wine-tasting events. The wines were anonymized, labeled with colors and numbers, and each participant sampled a combination of wines.
Professor Serge Belongie, a co-author from the Department of Computer Science and head of the EUU's Pioneer Centre for AI, adds:
"We have observed that when the algorithm combines data from wine labels, reviews, and wine tastings, it makes more accurate predictions about people's wine preferences than when it relies solely on traditional data in the form of images and text. Teaching machines to harness human sensory experiences results in superior algorithms that benefit users."
Applications Beyond Wine
Serge Belongie highlights the growing trend in machine learning of using multimodal data, typically consisting of a combination of images, text, and sound. The incorporation of taste and other sensory inputs is groundbreaking and holds immense potential, especially in the food sector.
"Understanding taste is a crucial aspect of food science, vital for achieving healthy and sustainable food production. However, the use of AI in this context is still in its infancy. This project illustrates the power of integrating human-based inputs into artificial intelligence, and I anticipate that these results will stimulate further research at the intersection of food science and AI," says Belongie.
Emma Andersen emphasizes that their method can be effortlessly adapted to other types of food and beverages:
"While we chose wine as a case study, the same approach can be applied to beer and coffee, among others. This method can be used to recommend products and potentially even food recipes to individuals. By better understanding taste similarities in food, we can also employ this technology in the healthcare sector to create meals tailored to patients' tastes and nutritional requirements. It may even lead to the development of foods tailored to different taste profiles."
The researchers have openly shared their data, which is available for free use by others in the field.
"We hope that individuals and organizations will find value in our dataset and use it to advance their own work. We have already received requests from people interested in contributing additional data to our dataset, which is truly exciting," concludes Emma Andersen.
Last updated: 2023-03-17